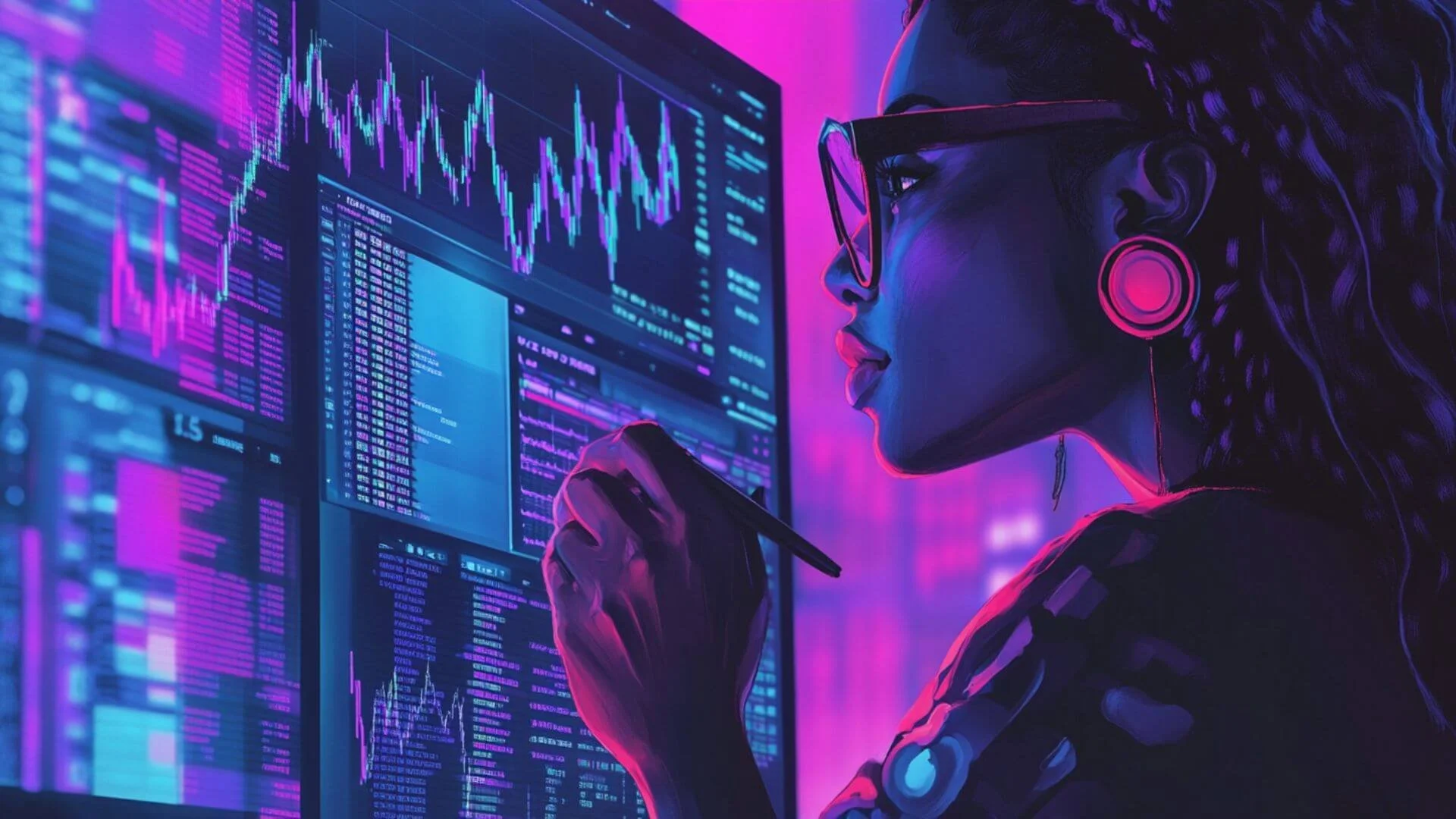
AI Adoption in Finance Is Stalling. Here’s What Needs to Change…
Unpack the real reasons AI adoption is stalling in finance: talent gaps, compliance pressure, legacy tech - and why most hiring strategies miss the mark. Discover how a smarter, market-specific approach unlocks AI that actually delivers!
Published April 2025
By Caspian One - AI Practice
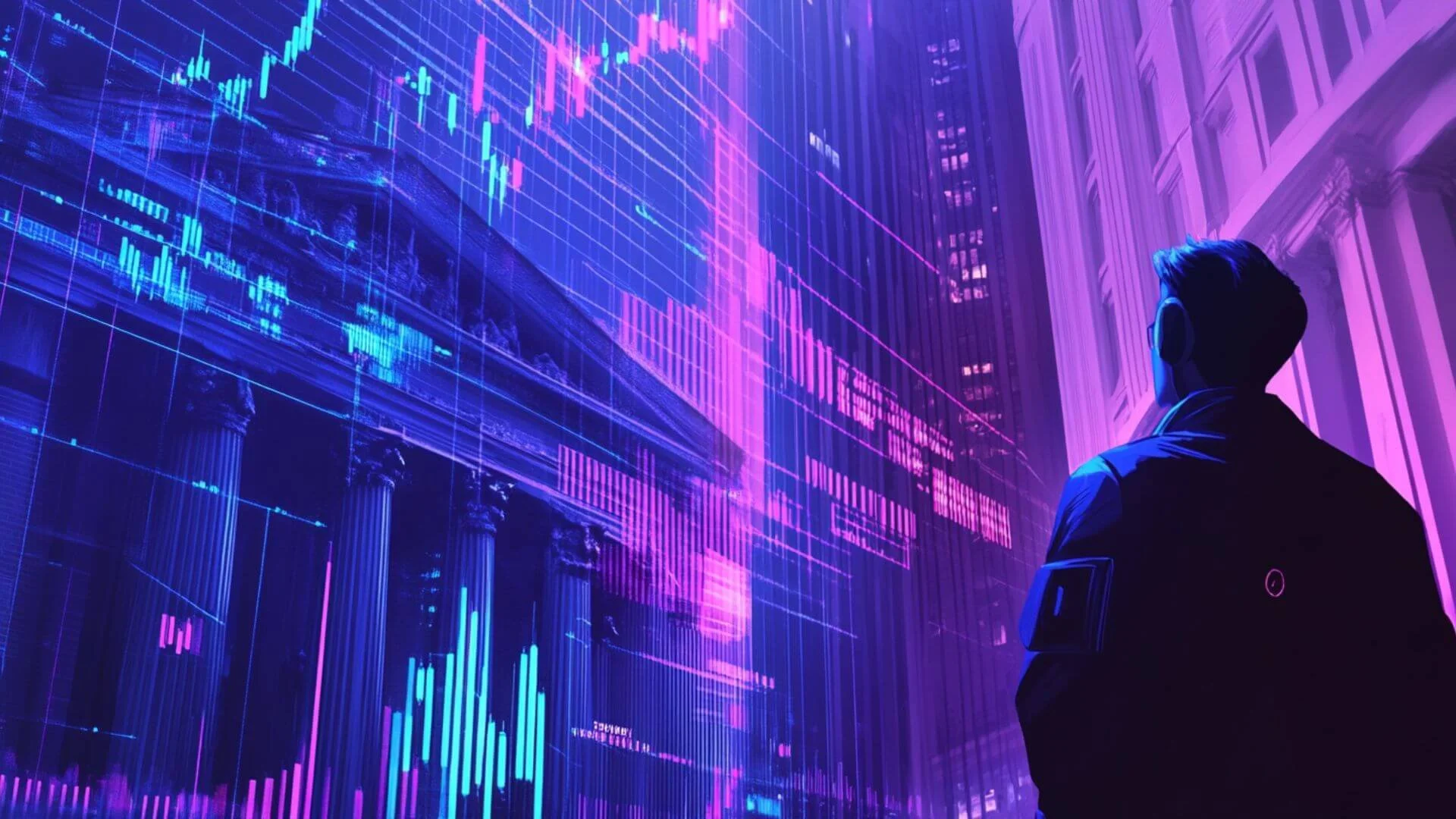
[Executive Brief]
Artificial Intelligence (AI) is rapidly transforming financial services, but the majority of institutions are struggling to turn promise into performance.
Despite increased investment and board-level urgency, AI adoption at scale remains slow, expensive, and underwhelming in terms of ROI.
The underlying issue isn’t the technology - it’s the talent.
This research explores the true state of AI adoption in financial markets, spotlighting the systemic barriers that prevent progress: talent shortages, regulatory pressure, legacy systems, and cultural inertia.
Crucially, we examine why traditional AI hiring models often fail in finance, and how a smarter, industry-specific approach to talent can unlock scalable, compliant, and high-impact AI solutions.
Grounded in learnings from McKinsey, BCG, Deloitte, EY, PwC, and others, and supported by insight from Freya Scammells, AI Practice Lead at Caspian One, this paper offers a practical roadmap for financial firms looking to future-proof their AI capability through better-aligned talent strategies.
The Real Reason AI Projects Fail…
AI is no longer a futuristic potential - it’s a boardroom priority.
By late 2025, over 70% of financial institutions will be utilising AI at scale, up from just 30% in 2023 (Gartner). From algorithmic trading and fraud detection to risk modelling and compliance automation, AI has the potential to enhance decision-making, improve efficiency, and reduce cost across virtually every area of the business.
Yet for all the promise, reality has fallen short.
According to Deloitte’s Financial AI Adoption Report (2024), only 38% of AI projects in finance meet or exceed ROI expectations; Over 60% of firms report significant implementation delays.
What’s going wrong?
At Caspian One, we believe the answer is clear: most financial institutions are hiring the wrong people.
This whitepaper unpacks that disconnect, using real data and market examples to help institutions understand where and why their AI strategies are stalling - and what they can do to change that.
“It’s not a question of whether AI can deliver value - it’s whether you have the right people who can deliver AI in your world. That means people who understand both the technology and the regulatory, operational, and cultural realities of finance.”
- Freya Scammells, Head of Caspian One’s AI Practice > contact
[Adoption Trends and Barriers]
A Market in Acceleration
Investment in AI across financial services has surged. McKinsey’s Global AI Survey (2024) reported that 58% of financial institutions directly attribute revenue growth to AI - primarily through enhanced trading performance, predictive risk management, and automation of operational processes.
AI-enabled fraud detection is already making a measurable difference.
Projections suggest that AI-based fraud systems will save global banks over £9.6 billion annually by 2026. Banks using advanced AI models report fraud detection accuracy exceeding 90%, reducing operational loss and boosting consumer confidence.
At the same time, the commercial viability of AI is clear: BCG (2024) notes that institutions adopting AI with specialist teams see up to 60% efficiency gains and 40% cost reductions in areas such as onboarding, compliance, and settlement.
The overall AI market in finance is projected to grow from £28.93 billion in 2024 to £143.56 billion by 2030, reflecting a compound annual growth rate (CAGR) of 30.6%. Within that, generative AI - a fast-emerging subset focused on content creation, automation, and data synthesis - is expected to grow even faster, rising from £1.67 billion to £12.10 billion over the same period, at a CAGR of 39.1%.
But Most AI Projects Still Underperform
Despite these promising headline figures, deeper analysis reveals significant challenges:
Only 29% of financial institutions report that AI has delivered meaningful cost savings, indicating many initiatives still fail to achieve significant operational efficiency (Boston Consulting Group, 2024)
65% of financial institutions experience implementation delays averaging 14 months, primarily driven by shortages in specialised AI talent who understand the intricacies of the financial sector (EY, Financial Services CTO Survey, 2024)
Institutions leveraging finance-specialised AI talent report significantly higher success rates and ROI -
with domain-experienced AI specialists achieving implementation nearly 80% faster than generalist counterparts (Goldman Sachs, AI Talent Insights, 2024).
The lesson is stark: general AI investment doesn’t guarantee AI success. The differentiator is the type of talent embedded in these initiatives.
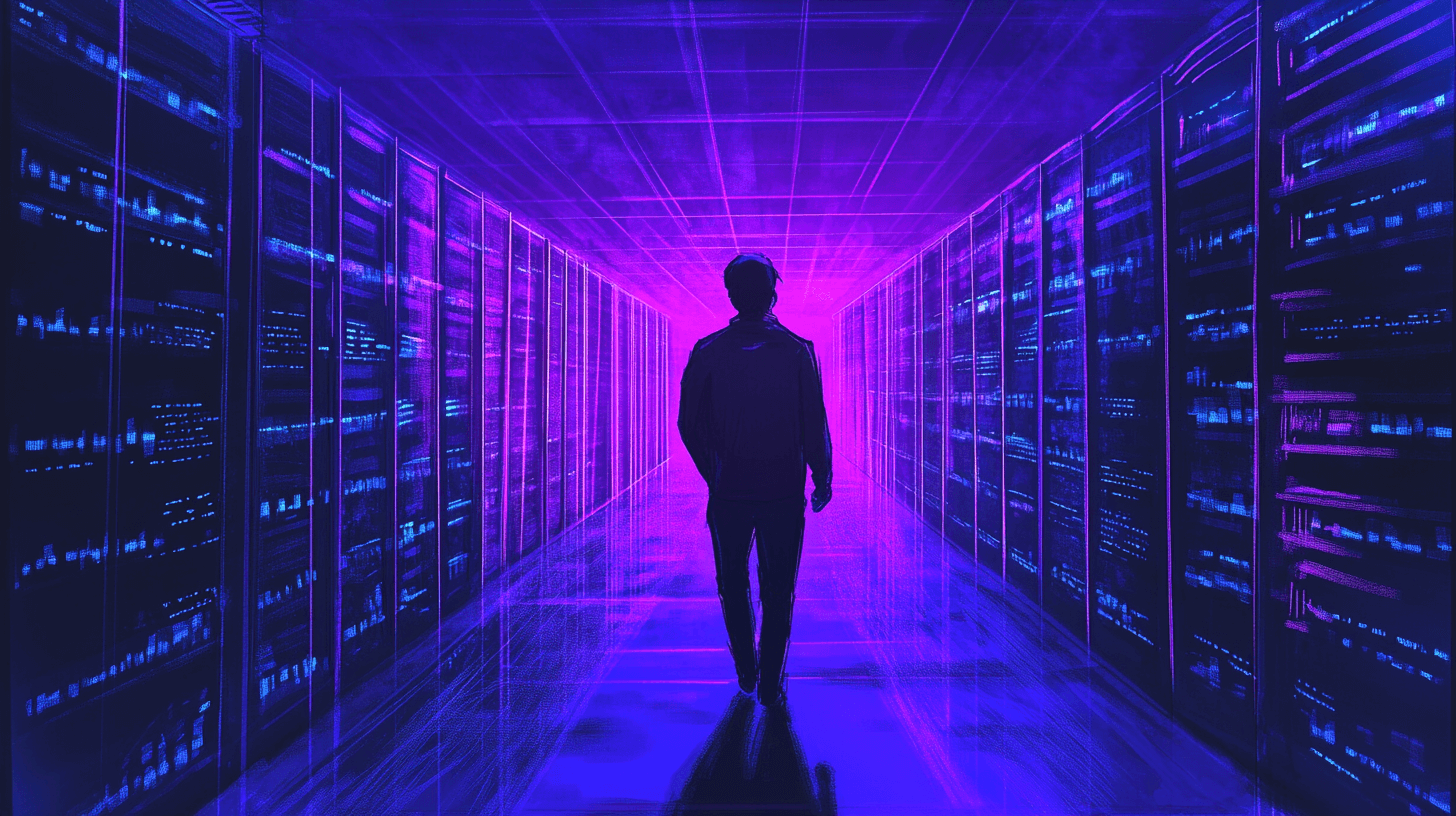
Why Traditional AI Hiring Fails in Finance: The Generalist Problem
There’s a growing tendency to hire highly technical machine learning (ML) engineers from big tech or research backgrounds. While these individuals excel in model-building, many lack understanding of financial systems, compliance mandates, or operational constraints.
Goldman Sachs (2024) reinforces this point:
“We found that AI specialists familiar with finance produced successful outcomes 79% faster than generalists. This difference translates directly to millions in saved investment and faster realisation of returns.”
“We’ve seen countless projects stall because firms hired AI experimenters - not implementers. The talent gap isn’t just technical - it’s contextual.”
- Freya Scammells, Head of Caspian One’s AI Practice > contact
Here’s what typically happens when teams hire for technical skill without sector alignment.
2.
Projects are delayed by compliance rewrites and legal bottlenecks
4.
Infrastructure isn’t designed for low-latency, real-time financial environments
1.
AI models are built to optimise accuracy - not regulatory explainability
3.
ML teams don’t understand trading logic, portfolio constraints, or real-world risk
The result?
High-cost initiatives that never make it out of the lab.
[Barrier 1]
Specialist Talent Shortages
The World Economic Forum (2024) reports that 73% of financial services leaders cite AI talent scarcity as a critical barrier to progress. However, this isn’t just a case of not having enough people - it’s a matter of not having the right kind of people.
What financial institutions need are not just highly skilled technologists, but professionals who understand the nuance of applying AI within complex, regulated, and high-stakes environments. The roles most acutely affected include:
-
These professionals are responsible for developing the core algorithms that drive AI solutions across trading, risk, and operational platforms.
In financial services, their work requires more than theoretical knowledge - it demands fluency in building low-latency, high-throughput models that operate in real time and within tightly governed environments.
They must optimise for performance under constraints such as market volatility, regulatory limits, and risk exposure. Familiarity with trading architecture, time-series data, and algorithmic model tuning is essential.
-
Often sitting at the intersection of quant finance and machine learning, these experts design and refine models that drive predictive analytics, portfolio optimisation, and alpha generation.
They apply advanced statistical techniques, deep learning, and reinforcement learning methods, but always within the context of financial markets.
Their domain knowledge allows them to assess model outputs not just for accuracy, but for economic significance, interpretability, and regulatory compliance. This role is vital in ensuring that AI adds meaningful business value, not just technical novelty.
-
MLOps (Machine Learning Operations) specialists ensure that AI systems are not only built, but deployed, monitored, and maintained at scale.
In finance, this means integrating models into production environments where uptime, traceability, and explainability are non-negotiable. MLOps engineers build and manage robust pipelines for data ingestion, model versioning, and real-time monitoring.
They also play a critical role in governance - making sure that AI models perform consistently, don’t drift, and remain compliant with evolving internal and external controls.
-
Natural Language Processing is increasingly central to financial services - powering everything from regulatory compliance automation and contract analysis, to market sentiment analysis and AI assistants for internal operations.
NLP specialists in this space require more than language model expertise - they need an understanding of financial language, documentation formats, and the implications of extracting insights from sensitive or regulated content.
Their work directly impacts risk mitigation, reporting accuracy, and client communications.
-
As the regulatory landscape tightens, institutions must design AI systems that are not just effective - but also explainable, auditable, and fair. AI governance professionals bring expertise in compliance frameworks such as the EU AI Act, SEC guidance, and FCA rules.
They work closely with risk, legal, and compliance teams to ensure that model development adheres to ethical standards and avoids reputational, operational, or legal risk.
Their role is increasingly central in helping firms establish trust in AI across internal stakeholders and external regulators alike.
[Barrier 2]
Regulatory Complexity and Compliance Risk
As artificial intelligence becomes more embedded in financial decision-making, regulators across the globe are moving quickly to ensure that these systems are safe, fair, and accountable.
The forthcoming EU AI Act (2025) is the most comprehensive regulatory framework to date, and its implications for financial services are far-reaching. Under the Act, firms deploying high-risk AI systems - such as those involved in trading, credit scoring, or fraud detection - must comply with strict obligations around transparency, documentation, risk mitigation, and human oversight. Non-compliance could result in penalties of up to 6% of global annual turnover, posing a significant financial and reputational risk.
Alongside the EU, the SEC has issued new guidance focused on the use of AI in investment advice, algorithmic trading, and client communications - placing greater emphasis on the explainability and auditability of automated decisions. In the UK, the FCA has increased scrutiny of algorithmic trading platforms and AI-driven risk models, particularly those that impact market integrity or consumer outcomes.
In this environment, financial institutions are no longer simply encouraged - but increasingly obliged - to ensure their AI systems are:
Transparent & Explainable:
Capable of being understood by stakeholders, including regulators, clients, and internal governance teams. Black-box models with opaque decision logic are increasingly untenable in high-risk contexts.
Auditable:
Institutions must be able to evidence how decisions were made, what data was used, and whether appropriate controls were in place. This demands traceable workflows, version-controlled models, and structured governance processes.
Bias-Free & Fair:
Regulators expect firms to identify, monitor, and mitigate algorithmic bias. This includes regular fairness audits, scenario testing, and alignment with ethical AI frameworks.
Traceable & Accountable:
Every component of an AI system - data sources, model architecture, performance metrics, and decision logs - must be documented and accessible for audit and oversight purposes.
This complexity means compliance cannot be treated as an afterthought.
Instead, it needs to be integrated from the ground up, embedded into hiring, infrastructure, and development processes.
As PwC (2024) puts it:
“AI compliance isn’t optional. Institutions require governance specialists who understand both the models and the laws that govern them.”
Many firms are now recognising that the lack of AI governance talent is as much a barrier to AI adoption as infrastructure or investment. Without experienced professionals capable of aligning innovation with regulatory expectations, AI projects risk becoming not just ineffective - but non-compliant and unrealisable.
[Barrier 3]
Legacy Infrastructure and Technical Debt
While AI is often viewed as a cutting-edge solution, its success is intrinsically tied to the maturity of the environment it operates in. In financial services - particularly in established Tier 1 banks - legacy infrastructure remains one of the most significant barriers to scalable AI adoption.
Modern AI systems depend on:
Cloud-native architectures capable of supporting distributed training and scalable deployment
High-throughput data pipelines to manage the volume and velocity of financial data
Real-time feedback loops to facilitate continuous learning, monitoring, and model refinement
Yet many institutions are still working within ecosystems built a decade - or more - ago.
Core platforms often consist of monolithic applications, tightly coupled data sources, and outdated technology stacks that lack the flexibility needed for AI integration. According to EY’s Financial Services CTO Survey (2024):
68% of CTOs cited legacy systems as the most significant obstacle to AI adoption
AI initiatives commonly experience delays of 12–18 months due to compatibility challenges with existing infrastructure
Projects that reach deployment often do so with limited scalability or automation, undermining their long-term value
“AI can’t create value in isolation. If it can’t plug into your architecture, run in real time, or feed back into your business systems, it remains an academic exercise.”
- Freya Scammells, Head of Caspian One’s AI Practice > contact
The Hidden Cost of Technical Debt
Legacy environments create a cascade of operational and strategic challenges. Data silos limit access to clean, structured, and relevant data required for model training. Manual workflows inhibit the integration of AI into existing processes. Security and compliance concerns delay cloud migration and AI tool adoption. Lack of observability and automation increases the cost of model monitoring, versioning, and governance.
Even where AI pilots are successful in isolated test environments, institutions often struggle to transition from proof-of-concept to production due to friction with existing systems.
Modernisation without disruption: addressing legacy challenges doesn’t necessarily require a wholesale rebuild. Many institutions are adopting incremental strategies, such as:
Deploying AI solutions in containerised or hybrid-cloud environments
Using middleware and orchestration tools to bridge old and new systems
Building modular AI components that can integrate with existing processes via APIs
Phasing cloud migration to minimise operational risk while improving flexibility
However, this transition requires both technical leadership and specialist AI infrastructure talent - professionals who understand not just the engineering, but how to navigate organisational complexity and risk sensitivity within financial institutions.
Without this, even the most sophisticated AI models are likely to remain stuck on the shelf - underused, untrusted, and ultimately, unscalable.
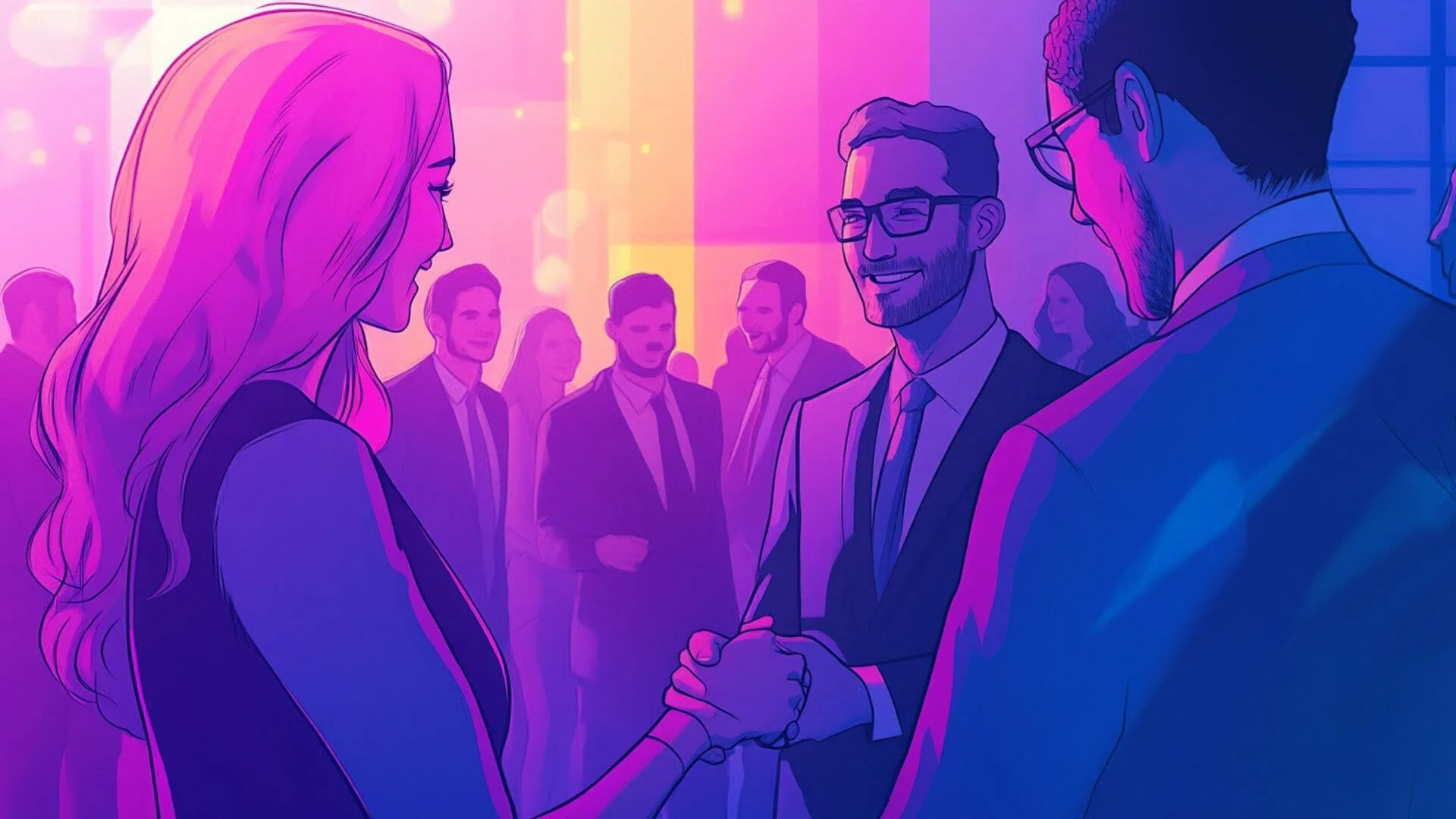
A Smarter Approach to AI Hiring in Finance
To overcome the systemic barriers outlined above, financial institutions must reframe how they approach AI capability building. The solution isn’t simply to hire more - it’s to hire differently.
The firms seeing meaningful returns from AI are those that build blended teams with domain-specific expertise baked in from day one. According to research from McKinsey, Deloitte, and Goldman Sachs, successful AI transformation in finance hinges on three specialist profiles.
Caspian One’s AI Practice is built around precisely these needs, curating a network of highly specialised professionals with both technical depth and financial fluency.
Would you prefer to speak directly with our AI Lead about smarter AI hiring?
If you’re navigating the complexities of building or scaling AI teams in financial services, sometimes the most efficient next step is a conversation.
Freya, our AI Lead, works closely with financial institutions to solve real-world talent challenges - balancing technical expertise with sector-specific insight. Whether you’re at the early stages of adoption or refining an established strategy, she’s available to explore how a more intelligent, commercially aligned acquisition model could work for you.
Financial AI Engineers
These are AI practitioners fluent in finance - not just Python.
They understand trading desks, portfolio theory, and risk controls. They build models that can survive scrutiny from compliance teams and work within the real-world constraints of latency, capital requirements, and regulatory boundaries.
“You don’t want someone learning what a swap is halfway through your quant project.”
MLOps & AI Infrastructure Specialists
Without MLOps, even the best models will fail in production.
These professionals:
Design CI/CD pipelines for machine learning
Maintain real-time monitoring and feedback loops
Ensure reproducibility, scalability, and fault tolerance in financial systems
Firms investing in MLOps experience significantly shorter deployment cycles and greater model reliability (EY, 2024).
“AI isn’t just a science problem - it’s an engineering one. If you can’t deploy it, you can’t scale it.”
AI Governance & Compliance Experts
With regulations tightening globally, firms need professionals who can embed responsible AI practices at every stage of the pipeline - model design, deployment, monitoring, and audit.
These specialists understand how to:
Detect and mitigate bias
Ensure explainability for high-risk use cases
Align AI pipelines with FCA, SEC, and EU AI Act standards
According to PwC (2024), early-stage involvement of compliance experts can reduce the likelihood of regulatory breaches by over 70%.
Strategic Recommendations and Conclusion
Financial institutions are no longer debating whether to invest in AI - they’re now grappling with how to make that investment pay off. The key lies not in technology alone, but in who is trusted to design, build, and scale these solutions.
Here’s how institutions can shift towards AI strategies that deliver sustained, measurable value:
1. Prioritise Sector-Specific Talent
Generic AI expertise is no longer sufficient. Financial services firms should prioritise:
Hiring AI professionals with direct experience in finance
Seeking cross-functional understanding of both technical and regulatory constraints
Embedding domain knowledge into every stage of the AI lifecycle
This alignment improves time-to-value, model performance, and stakeholder confidence.
2. Integrate Governance from Day One
Don’t wait until deployment to think about compliance. Embed AI governance from the outset by:
Employing specialists with regulatory and ethical AI experience
Ensuring all models are explainable, auditable, and compliant
Aligning model outputs with industry-specific transparency standards
This reduces risk, accelerates approval processes, and builds internal trust in AI.
3. Build Infrastructure That Supports Scale
AI success isn’t only about talent - it also requires systems that can support deployment at scale. That means:
Investing in cloud-native tools and scalable data platforms
Breaking down legacy silos that slow down model integration
Hiring MLOps experts to operationalise AI workflows securely and efficiently
Without the right infrastructure, even world-class talent can’t deliver sustainable AI adoption.
4. Rethink the Role of Experimentation
Innovation is vital, but finance is not a research lab. Many firms fall into the trap of endless pilots that never translate into production. The smarter approach:
Define clear business outcomes before model development
Focus on practical applications with measurable ROI
Employ talent capable of bridging strategy and execution
“The AI conversation in finance needs to shift from possibility to practicality. That starts with hiring people who know how to make AI work - not just make it interesting.”
- Freya Scammells, Head of Caspian One’s AI Practice > contact
In conclusion…
AI is undeniably reshaping financial services - but turning that potential into real-world results hinges on one critical factor: the right talent.
Institutions that continue relying on generalist AI hires or research-heavy teams often find themselves facing prolonged delays, rising costs, and mounting compliance risk.
By contrast, firms that prioritise finance-specific AI expertise - practitioners who understand both the technology and the regulatory and operational realities of the sector - are able to move faster, embed trust, and realise measurable value.
Caspian One’s dedicated AI Practice was built with this in mind.
Led by industry specialist Freya Scammells, the practice addresses the core capability gaps most institutions face today - from AI governance and MLOps to financial engineering and real-time model deployment.
Every professional in our network combines technical depth with domain fluency, ensuring AI is not just delivered but delivered effectively, securely, and in line with business and regulatory priorities.
As the pace of AI adoption accelerates, the institutions that lead will be those who build teams capable of executing with precision, speed, and accountability. Strategic partnerships with specialist providers - those who understand the nuances of both AI and finance - are becoming not just advantageous, but essential.
Disclaimer
This report is based on research from credible, publicly available sources and Caspian One’s internal market expertise as of the time of writing. While every effort has been made to ensure the accuracy, reliability, and completeness of the content, this document is intended for informational purposes only and should not be interpreted as formal advice or a specific recommendation regarding AI adoption or hiring strategies.
The AI landscape - along with the associated regulatory frameworks and talent markets within financial services - is developing rapidly. Readers are encouraged to independently verify current standards, regulations, and market conditions before making strategic decisions or investments. Caspian One accepts no liability for any actions taken based on the information provided herein.
Any case studies, scenarios, or ROI figures included in this report are illustrative and do not represent guaranteed outcomes. Organisations should conduct their own due diligence and seek relevant professional guidance before implementing any AI-related initiatives. For consistency all currencies have been converted to UK GBP with exchange rates as-of April 2025.
This report is the intellectual property of Caspian One and was produced and published in March 2025. All rights reserved.
-
AI Practice at Caspian One (2025)
Caspian One AI Practice: Specialist AI Talent for Financial Markets.
https://www.caspianone.com/ai-practice
Accenture (2024)
Accenture Financial AI Report 2024: Maximizing Value from AI Investments in Banking and Capital Markets.
https://www.accenture.com/financial-ai-report-2024
Boston Consulting Group (2024)
AI in Financial Services: Unlocking Efficiency and Value through Specialized Talent.
https://www.bcg.com/publications/2024/ai-financial-services-specialist-talent
Deloitte (2024)
Financial AI Adoption Report 2024: Expectations vs. Reality.
https://www.deloitte.com/financial-ai-adoption-report-2024
EY (2024)
Financial Services CTO Survey 2024: AI Integration and Legacy System Challenges.
https://www.ey.com/financial-services-cto-survey-2024
Gartner (2023-2025)
Gartner Hype Cycle for Artificial Intelligence in Banking and Investment Services, 2024.
https://www.gartner.com/ai-hype-cycle-banking-2024
Goldman Sachs (2024)
Goldman Sachs AI & Talent Market Insights Report, 2024.
https://www.goldmansachs.com/insights/ai-talent-market-2024
LinkedIn Talent Insights (2024)
Financial Sector AI Talent Trends Report 2024.
https://business.linkedin.com/talent-solutions/ai-talent-trends-2024
McKinsey & Company (2024)
Global AI Survey: State of AI in Financial Services 2024.
https://www.mckinsey.com/business-functions/mckinsey-digital/global-ai-survey-2024
PwC (2024)
Navigating AI Compliance: A Guide to Upcoming Regulations in Financial Services.
https://www.pwc.com/financial-ai-compliance-guide-2024
World Economic Forum (WEF, 2024)
The Future of Jobs Report 2024: AI Talent and Skill Gaps in Finance.
-
Capgemini Research Institute (2024)
AI in Capital Markets: Accelerating Digital Transformation.
https://www.capgemini.com/ai-capital-markets-2024
CFA Institute (2024)
AI and Data Science in Investment Management: Opportunities and Challenges.
https://www.cfainstitute.org/research/ai-data-science-investment-2024
European Commission (2024)
EU AI Act Documentation: Guidelines, Compliance, and Implementation.
https://ec.europa.eu/digital-strategy/ai-act-2024
IBM Institute for Business Value (2024)
The State of AI in Banking and Financial Markets.
https://www.ibm.com/business/value/ai-financial-markets-2024
KPMG (2024)
Artificial Intelligence Regulatory Horizon Report 2024.
https://home.kpmg/ai-regulatory-report-2024
Oliver Wyman (2024)
AI in Financial Risk Management: Driving Value from Machine Learning.
https://www.oliverwyman.com/ai-financial-risk-2024
SEC.gov (2024)
SEC Guidelines for AI-Driven Trading and Investment Advisory (2024 update).